Why MLSecOps Should be Central to Your Cybersecurity Strategy
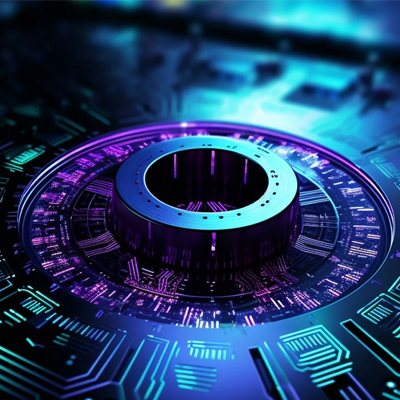
As organizations increasingly adopt AI and Machine Learning (ML) to streamline operations and enhance competitiveness, ensuring the security of these technologies has become critical. A key concern is the integrity of the data used to train ML models, as corrupted data can lead to unpredictable behavior.
The rise of ML has also seen an increase in backdoor attacks, where hackers use data poisoning techniques to compromise ML models with harmful data. Once triggered, these attacks can have devastating consequences. For instance, the “Sleepy Pickle” technique exploits the insecure Pickle file format, targeting ML models at the distribution stage and embedding vulnerabilities that attackers can later exploit.
Given these growing risks, organizations must integrate MLSecOps into their cybersecurity strategy, focusing on safeguarding the foundational stages of ML model development and ensuring that robust security measures are in place throughout the lifecycle.